Air Pulse – An Air Quality Status Analysis Model
Keywords:
Air Quality Prediction, Air Quality Index (AQI), Machine Learning Algorithms, Environmental Pollution, Central Pollution Control BoardAbstract
A global concern is scaling due to the rapid growth of air pollution. Air Pollution is a combination of various gases in the air with the presence of countless solid particles. To protect human life and the deteriorating situation of air quality that is severely affecting the flora and fauna, we aim to design and train a system to predict the quality of the air that surrounds us with the utmost accuracy and reliability. The Air Quality Index of India is a standard computation used to specify the extent of impurities over an interval. India is impacted severely as it hosts 22 of the world’s 30 extremely populated cities. We have used and contrasted the machine learning algorithms to achieve the prime results. Interjection, feature analysis, and prediction are three major factors in inner-city air computing. Considering this research, multiple processing methods are used to process the data. Our model will have the capacity to predict the status of the air quality in different parts of the Delhi. The model provides almost 85% accuracy by outlining the correct status of the quality of air in different regions of Delhi. This system can be referred to by the Central Pollution Control Board to understand whether the air is safe or unsafe and record the air quality along with the congregation of defiled particles. The user interface will act as a one-stop platform to track and get updates about the air quality of different regions of the national capital, Delhi.References
[1] Maltare, N.N. and Vahora, S., 2023. Air Quality Index prediction using machine learning for Ahmedabad city. Digital Chemical Engineering, 7, p.100093.
[2] Bali, S. and Sengar, M.N., 2020. Indian air quality prediction and analysis using machine learning. J Eng Sci, 11(5), pp.554-557.
[3] Gupta, N.S., Mohta, Y., Heda, K., Armaan, R., Valarmathi, B. and Arulkumaran, G., 2023. Prediction of Air Quality Index Using Machine Learning Techniques: A Comparative Analysis. Journal of Environmental and Public Health, 2023.
[4] Essamlali, I., Nhaila, H. and El Khaili, M., 2024. Supervised Machine Learning Approaches for Predicting Key Pollutants and for the Sustainable Enhancement of Urban Air Quality: A Systematic Review. Sustainability, 16(3), p.976.
[5] Mampitiya, L., Rathnayake, N., Leon, L.P., Mandala, V., Azamathulla, H.M., Shelton, S., Hoshino, Y. and Rathnayake, U., 2023. Machine learning techniques to predict the air quality using meteorological data in two urban areas in Sri Lanka. Environments, 10(8), p.141.
[6] Méndez, M., Merayo, M.G. and Núñez, M., 2023. Machine learning algorithms to forecast air quality: a survey. Artificial Intelligence Review, pp.1-36.
[7] Murukonda, V.S.N.M. and Gogineni, A.C., 2022. Prediction of Air Quality Index Using Supervised Machine Learning.
[8] Shankar, L. and Arasu, K., 2023. Deep Learning Techniques for Air Quality Prediction: A Focus on PM2. 5 and Periodicity. Migration Letters, 20(S13), pp.468-484
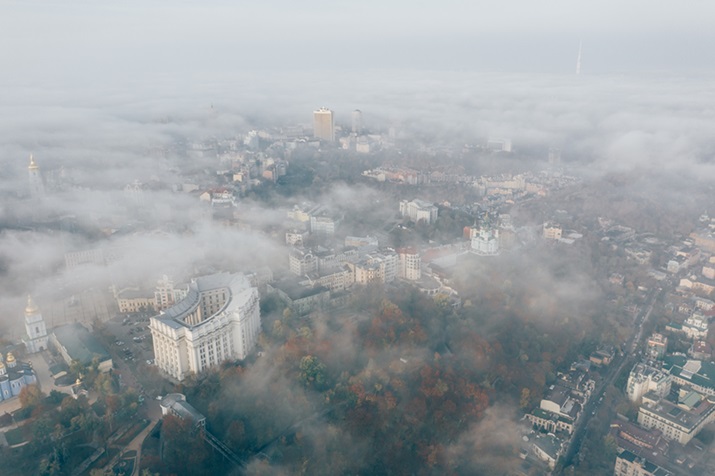
Downloads
Published
How to Cite
Issue
Section
Categories
License
Copyright (c) 2024 Prabhjot Kaur, Kavya Jain, Inika

This work is licensed under a Creative Commons Attribution 4.0 International License.
All Articles in 'International Research Journal of Scientific Studies' are Open Access articles published under the Creative Commons CC BY License Creative Commons Attribution 4.0 International License http://creativecommons.org/licenses/by/4.0/.
This license allows you to share – copy and redistribute the material in any medium or format. Adapt – remix, transform, and build upon the material for any purpose, even commercially.